Can Tech Save Us From Deepfakes?
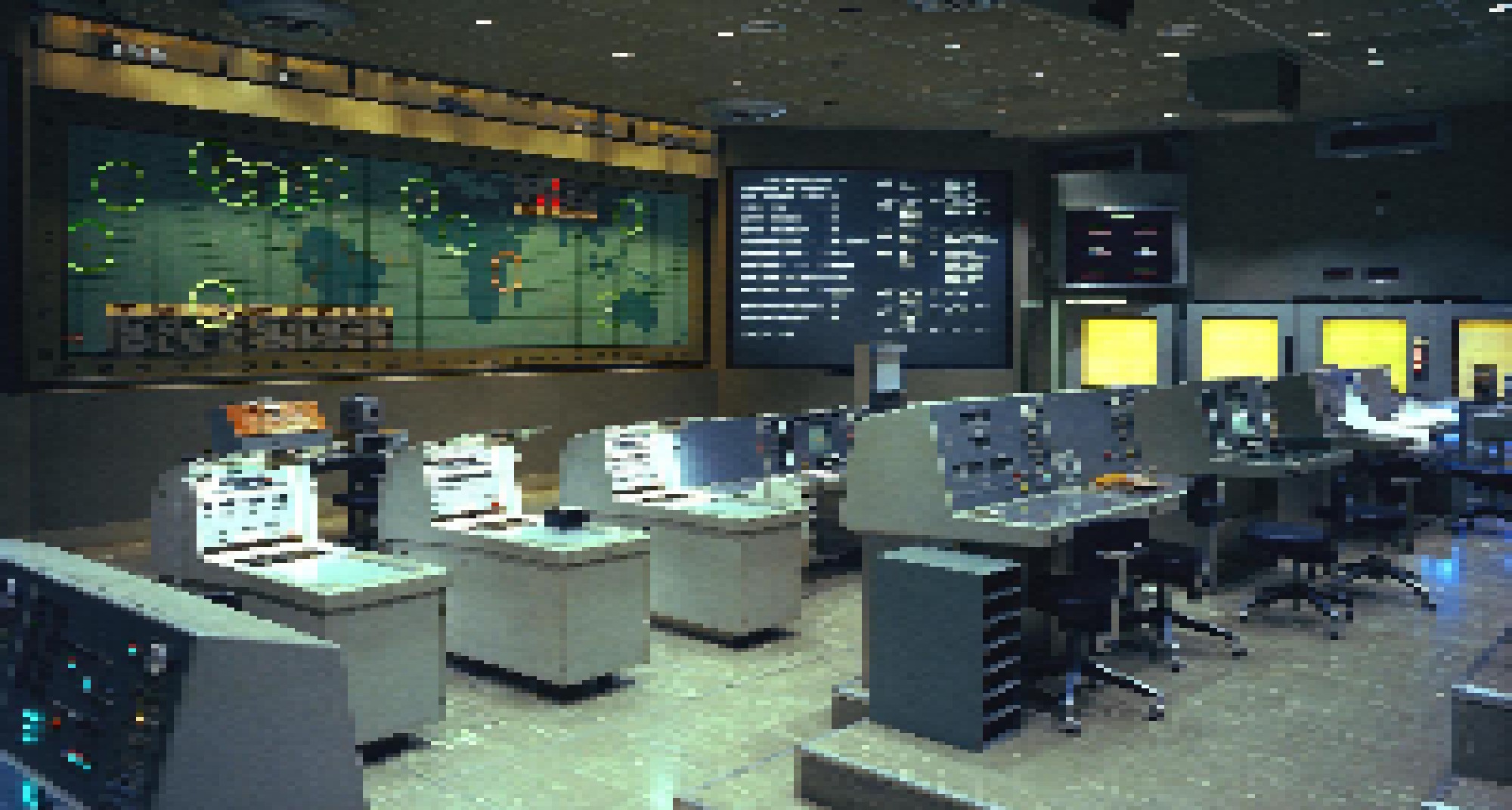
By: Pakinam Amer
Deepfakes are better and more pervasive than ever. A complex form of manipulated media generated by artificial intelligence software, these videos rely not only on realistic imagery but also perfectly synthesized human speech.
Even Disney is investing in deep learning-driven technology. In a paper published in early 2020, scientists from Disney Research Studios claimed that they had successfully trained an algorithm to render photo-realistic face-swapping in images and videos in unprecedented high-resolution quality. The entertainment giant hopes to use such effects to portray an actor as younger or to employ when an actor isn’t available, among other uses, the authors write.
Robert De Niro was “de-aged” for flashback scenes in the recent Netflix production of “The Irishman” (Credit: Lucasfilm, Ltd.)
But even as such technology goes mainstream, unresolved anxieties surround its use. From the moment deepfake videos started popping up across the web in the form of parodies, political satire, and fake celebrity porn, they have been met by equal measures of fascination and fear. A report released last year by AI company Deeptrace Labs estimates there are now around 49,000 deepfakes in circulation, but the rapid growth of face-swapping filters across social platforms and applications powered by facial recognition engines mean there are likely thousands more.
Artists and journalists have recently been exploring ways in which they can use the technology constructively to create social and political commentary, or to guard the privacy of marginalized and at-risk groups. For example, Academy-award-nominated documentary filmmaker David France used deepfake technology to mask the identities of gay and lesbian Chechens in his revelatory film Welcome to Chechneya, which was released last week on HBO. The technology was even used as a “fix-it” by a Star Wars superfan to improve a cameo of young Princess Leia in Star Wars: Rogue One.
But even as individuals and massive media companies like Disney harness this technology for non-malicious purposes, deepfake technology is engendering fierce debate over fake news, individual privacy, and the weight of video evidence.
The founder of YouTube channel Derpfakes used deepfake technology to improve the appearance of a younger Carrie Fisher in Star Wars: Rogue One. The project, which was viewed thousands of times within the first few days, was done “in the time it takes to watch an episode of The Simpsons”, he told The Guardian.
The challenge of detection
In January, Facebook announced that it would ban manipulated media that distorts reality or misleads the public, possibly to nip the problem in the bud before the 2020 elections.
This includes videos “edited or synthesized beyond adjustments for clarity or quality in ways that aren’t apparent to an average person and would likely mislead someone into thinking that a subject of the video said words that they did not actually say,” wrote Monika Bickert, VP of Global Policy Management at Facebook, in a blog post. She added that audio or video, whether deepfake or not, would also be governed by Facebook’s community standards, and would be removed if it included nudity, graphic violence, voter suppression, or hate speech.
This standard applies to ads as well, though a report by the New York Times suggested that this policy won’t be applied retroactively.
On the statewide level, California has preemptively banned the distribution of “malicious” manipulated videos, audio, and pictures that mimic real footage and intentionally falsify the words or actions of a political candidate within 60 days of an election. Marc Berman, the assemblyman who introduced the bill in reaction to a doctored Nancy Pelosi video that made the U.S. House Speaker appear drunk, called deepfakes “a powerful and dangerous new technology that can be weaponized to sow misinformation and discord among an already hyper-partisan electorate.”
An analysis by the Washington Post of the manipulated video of Nancy Pelosi which is slowed down making her appear to be slurring words. (Credit: Washington Post)
Last year the U.S. Senate passed the Deepfake Report Act, demanding the Department of Homeland Security launch an annual study of deepfakes and any AI-powered media that seeks to “undermine democracy.”
Paul Barrett, an adjunct professor of law at New York University, told NPR’s Troll Watch that disinformation is increasingly targeting images as opposed to text. Anyone with basic coding knowledge and “the desire to mess around with elections can begin to cobble together these very convincing but fake videos,” Barrett said. “And the companies are aware of this and are scrambling, perhaps belatedly, to try to respond to it.”
It’s too early to know whether legislation can be an effective strategy for stopping deepfakes from circulating or going viral, particularly given how many corners of the deep web are inaccessible to search engines. Another challenge is that such bills can potentially limit freedom of speech. So some experts are turning to another strategy of stemming the tide of deepfakes: fighting tech with tech.
Counter technology, to the rescue?
When it comes to spotting deepfakes, technologists and start-ups are playing catch-up. Some, however, are investing in developing future-proof methods to guard against the darker side of deepfakes.
In June, Facebook announced the results of a deepfake detection challenge that brought together more than 2,000 technologists and AI researchers to create and test algorithms to detect manipulated videos made by Facebook.
The top-performing model achieved 82.56 percent precision when tested against Facebook’s test dataset, but only a 65.18 percent accuracy rate when tested against a “blackbox” dataset containing real-world videos (which are typically previously unseen and more complex). Blackbox algorithms remain one of the biggest challenges facing machine learning detection technology.
Witness, an international nonprofit that uses video and technology-based strategies to expose corruption and aid human rights activism, has been lobbying for better investment in media forensics, citing the U.S. DARPA’s MediFor program as a promising step.
The Brookings Institute, a think tank in Washington, D.C., is urging policymakers to create an “an additional stream of funding awards for the development of new tools, such as reverse video search or blockchain-based verification systems, that may better persist in the face of undetectable deepfakes.” The institute also encourages policymakers to invest in training journalists and fact-checkers, and supporting collaboration with AI-based detection firms.
Deeptrace Labs is one such detection firm. A start-up that uses deep learning and computer vision to detect and monitor deepfakes, it promotes itself as “the antivirus” in the fight against viral AI-based synthetic videos — a not-too-subtle testament to the battle of wits at the frontiers of deep tech.
Deeptrace is developing analytical back-end systems that would detect fake videos, and could be used by individual users and media companies to help them recognize manipulation. “The tagline sums up quite well how we view some of the ways in which the problem is manifesting and how we see potential technological solutions to prevent it,” says Henry Ajder, head of communication and research analysis at Deeptrace Labs.
Reality Defender is another intelligent software that is built to run alongside you while you browse the web, detecting potentially fake media and alerting users to its presence.
Scientists are also part of the battle. Amit Roy-Chowdhury, a professor of electrical and computer engineering at the University of California, Riverside, and director of the Center for Research in Intelligent Systems, has developed a deep neural network architecture that can recognize altered images and identify forgeries with unprecedented precision.
Roy-Chowdhury’s system can tell the difference between manipulated images and unmanipulated ones by detecting the quality of boundaries around objects, down to the individual pixel. These boundaries can get “polluted” if the image has been altered or modified, and so can help researchers pinpoint where any doctoring has occurred.
While his system works with still images, in theory the same principle – with some adjustments – can be applied to deepfake videos, which consist of thousands of frames and images.
But despite solid efforts, most researchers agree that the process to detect deepfakes “in the wild” is a whole different ballgame. Plus, by and large, these experimental detection techniques are only in the hands of experts, and inaccessible to the general public.
Efforts by scientists and technologists to counter deepfakes with detection technology are growing stronger—but so are deepfakes themselves. (Credit: Francesca Panetta)
But technology can’t solve everything
“As our technology gets better, combating deepfakes will become increasingly difficult,” says Aleksander Madry, an associate professor of computer science at MIT whose research tackles key algorithmic challenges in computing and developing trustworthy AI. “So currently this is more of a cat-and-mouse game where one can try to detect them by identifying some artifacts, but then the adversaries can improve their methods to avoid these artifacts.”
“Better approaches may deceive the detection mechanism,” agrees Roy-Chowdhury. The computer scientist says it’s highly unlikely that “we’ll have a system which is able to detect each and every single deepfake. Typically, security systems are defined by the weakest link in the chain.”
According to Nick Roy, a professor at the MIT Computer Science & Artificial Intelligence Lab, scientists are often working in the dark. “In many cases, scientists have no idea what neural networks are doing,” says Roy, whose research into AI, machine learning, and computational systems is focused on the problems that result from uncertainty.
Scientists “can’t always interpret when something goes wrong. Neural networks are not black magic. We do understand a lot of the basics, but we don’t have our arms around the limits … we need to understand what the limits are,” he explains.
As the capabilities of deep-learning-based technology continue to evolve, Madry says that “my worry is that we will no longer be able to automatically rely on video as a proof.”
The future of deepfake technology and detection
For poorly generated deepfake videos—the kind with obvious wrinkles that typically result from crude editing—it’s easy for smart software and an informed eye to detect tampering. But as synthetic audio-visual media developers iron out the kinks, it will become exponentially more difficult to find telltale signs of forgery.
In previous years, researchers have trained software to spot deepfakes by monitoring frequency of blinking; humans blink an average of 17 times per minute, but older deepfakes often blinked far less frequently. “If you’re looking at a video from January 2018, the lack of blinking may well still apply there,” says Ajder. “But say in the next year, or two, or five, that’s not going to be reliable for measuring validity.”
Similarly, as deepfakes advance, detection tools that rely on training AI to account for editing glitches such as shifting frames and changes in lighting or skin tone may soon become obsolete.
Ajder says he’s confident that some, if not all, of what he refers to as “folk detection” methods of phony media “are almost certainly going to be trained out of advanced versions. They’re only useful if applied with caution and combined with analytic methods.”
Another problem is the sharing technologies used by social media platforms, which can allow deepfakes to multiply without detection.
For instance, Matthew Stamm, an assistant professor at Drexel University, said at South by Southwest last year that some detection techniques look for “really minute digital signatures” that are embedded in video files. But when a video file is shared on social media, it’s shrunken down and compressed, which removes all signs of tampering.
“There’s a lot of image and video authentication techniques that exist but one thing at which they all fail is at social media,” Stamm said.
Barrett of NYU Law, meanwhile, urges people to be “very skeptical about what they look at” and says companies should act aggressively against false information and perhaps “demote” those posts or videos. “I would argue that they ought to just take that kind of material off their sites altogether,” he adds.
Ultimately, combating the negative effects of altered videos, especially if they’re used to generate false news with an intent to mislead, will require new forms of collective action. The problem is pervasive enough that it can’t be solved alone: AI firms, media watchdogs, governments, and individuals must share the responsibility for fact-checking and deciding on what counts as truth and what doesn’t.
A version of this article appeared in print on 22 November 2019. The article has been revised and updated for online publication on 3 July 2020.
Lede photo credit: Source: NASA / Treatment: Halsey Burgund