Deepfakes 101
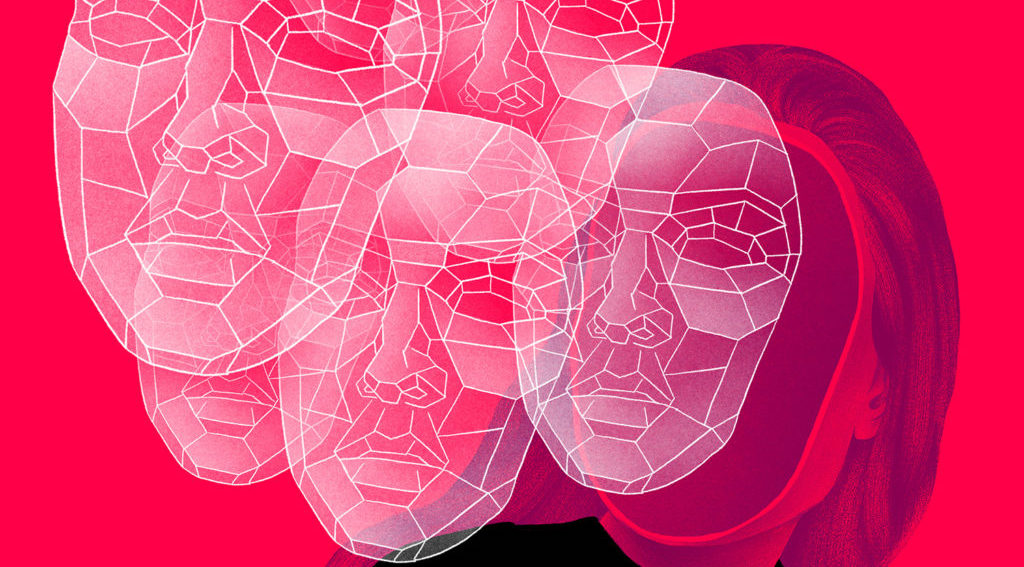
By: Joshua Glick
The word “Deepfake”—a combination of “deep learning” and “fake”—refers to any media that uses artificial intelligence to simulate people doing or saying things they never did or appearing in fabricated situations without their consent.
Deepfakes came into public consciousness in late 2017 with the proliferation of AI-manipulated face-swap videos across the Internet. Many of the most common examples involved celebrity heads attached to the bodies of adult film stars.[1] Deepfakes and the conversation around them have since moved from Reddit and 4chan to mainstream forums and social media platforms.
To be sure, there are many artistic and civic uses of synthetic media. AI can help create new forms of public history, provide innovative solutions to urban infrastructural challenges, and offer social critique. In Event of Moon Disaster itself, a multi-part installation and digital project, shows the creative potential of AI and warns against the dangers of disinformation. Deepfakes, however, are often geared towards more nefarious purposes – by far the most common use of deepfakes is pornography, where face-swapping constitutes a form of digital violence used to humiliate and harass. There is also potential for deepfakes to sow the seeds of confusion or to amplify “fake news.”
Media manipulation is a centuries-old phenomenon, spanning everything from spectacular hoaxes to deceptively edited films and photoshopped images.[2] As scholars Britt Paris and Joan Donovan argue, deepfakes are part of a wide spectrum of audio-visual media intended to dupe or confound viewers. Media that aims to mislead draws on a variety of techniques such as speeding up, slowing down, re-cutting (with new inserts), and re-contextualizing the original clip. “Cheapfakes” or “shallowfakes” refer to more low-tech, swiftly created media involving doctored images and distorted context.[3]
Four factors have contributed to the rise of deepfakes:
- advances in deep learning technologies and computational hardware
- the development of social media platforms that make it easier for people to share and access information
- a deregulated climate of media production and distribution
- the embattled state of contemporary journalism
Credible news organizations are forced to contend with outlets specializing in clickbait content, attacks against a free and independent press, and dwindling staff and resources.
To make the most common form of deepfake, the face-swap video, a creator replaces a “target face” with a “source face.” The idea is to give the impression that the source person was in fact performing the action depicted in the target person’s video. The creator first needs a “trained model” to make the swap, which involves algorithms processing a large data set of images related to the human face. This includes a wide range of facial features (eyes, ears, hair, etc.) as well as an equally wide range of particular qualities (tones, textures, etc.). The result is a trained model that not only possesses an inclusive understanding of the features of a human face, but the relationship between these features; for example, the positioning of the ear on the side of the head and the nose in the middle.
In this video, YouTube user ctrl shift face subtly morphs actor Bill Hader’s face into both Al Pacino and Arnold Schwarzenegger. (Credit: ctrl shift face)
Drawing on the training data, the AI begins a frame-by-frame reconstruction of the source person’s face in the context of the target person’s video. The person in the video retains the expressions, mannerisms and words of the target individual, but the facial features of the source—the person a viewer is supposed to think is doing the action. As a final step, post-production effects may be applied to the deepfake, to ensure that the movements of the head and neck look smooth and convincing.
There are numerous advanced techniques to heighten the quality of deepfakes. One of the most popular involves something called Generative Adversarial Networks. In this technique, both a generative and a discriminative algorithm learn from each other to produce a trained model. Whereas the latter tries to differentiate “real” versus “fake” iterations of the training data, the former tries to trick the discriminative network into accepting fake iterations as real. As the two algorithms go head to head, the generative algorithm eventually produces synthetic media that neither the discriminative algorithm (nor the human eye or ear) can easily tell is fake.
Politicians and celebrities are useful targets for deepfake creators, not just because they are so recognizable, but because there is plenty of available training data for an AI to build its model. Early examples included Barack Obama, Nicolas Cage, Mark Zuckerberg, Angela Merkel, Manoj Tiwari, Nancy Pelosi, Vladimir Putin, and Zulkifli “Zul” Ariffin.[4] According to a report from cybersecurity company Deeptrace Labs, there were close to 49,000 deepfake videos and 20 content creation hubs and websites online as of June 2020, and that number has continued to grow.[5]
In 2018, Jordan Peele’s Monkeypaw Productions, in collaboration with the news site BuzzFeed, issued a warning against the power of deepfakes—by making this deepfake video starring a synthesized President Barack Obama. (Credit: BuzzFeed Video)
Deepfakes have fast become a cultural phenomenon that has infiltrated the overlapping worlds of politics, entertainment, and journalism. They need to be examined as both a distinct form of synthetic media that draws on an evolving technological toolbox, as well as part of a broader landscape of disinformation.
Lede photo credit: Source: Joan Donovan and Britt Paris, Deepfakes and Cheap Fakes: The Manipulation of Audio and Visual Evidence, Data & Society, September 2019
[1] “Deepfake” gained notoriety around November 2017 by the Reddit user u/deepfakes, who subsequently created a deepfake forum dedicated to faceswapping female celebrities into pornography. Reddit subsequently banned the community. For coverage, see, for example, Adi Robertson, “Reddit bans ‘deepfakes’ AI Porn Communities,” The Verge, February 7, 2018.
[2] For this longer history, see, Kevin Young, Bunk: The Rise of Hoaxes, Humbug, Plagiarists, Phonies, Post-Facts, and Fake News (Minneapolis: Graywolf Press: 2017); Alexandra Juhasz and Jesse Lerner, eds. F is for Phony: Fake Documentary and Truth’s Undoing (Minneapolis: University of Minnesota Press, 2006).
[3] Joan Donovan and Britt Paris, “Beware the Cheapfakes,” Slate, June 12, 2019; Joan Donovan and Britt Paris, Deepfakes and Cheap Fakes: The Manipulation of Audio and Visual Evidence, Data & Society, September 2019; Sam Gregory, “Deepfakes Will Challenge Public Trust in What’s Real. Here’s How to Defuse Them,” Diffusing Disinfo, February 19, 2019.
[4] Samantha Cole, “AI-Assisted Fake Porn Is Here and We’re All Fucked,” Motherboard, December 11, 2017; Kevin Roose, “Here Come the Fake Videos, Too,” New York Times, March 4, 2018; Maheen Sadiq, “Real v Fake: Debunking the ‘drunk’ Nancy Pelosi Footage,” The Guardian, May 24, 2019; Allyson Chiu, “Facebook Wouldn’t Delete an Altered Video of Nancy Pelosi. What About One of Mark Zuckerberg?” Washington Post, June 12, 2019; Aja Romano, “Jordan Peele’s Simulated Obama PSA is a Double-Edged Warning Against Fake News,” Vox, April 18, 2018; Sheith Khidhir, “Malaysian Actor in ‘Porn’ Video Blames Deepfake,” The Asean Post, December 16, 2019; Sarah Cahlan, “How Misinformation Helped Spark an Attempted Coup in Gabon,” Washington Post, February 13, 2020.
[5] Henry Ajder et. al, The State of Deepfakes: Landscape, Threats, and Impact, Deeptrace Labs, September 2019; Henry Ajder, “Deepfake Threat Intelligence: A Statistics Snapshot from June 2020,” Deeptrace Labs, July 3, 2020.